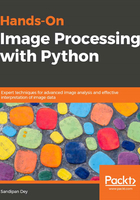
Why do we need the DFT?
First of all, transformation to the frequency domain leads to a better understanding of an image. As we'll see in the next few sections, the low frequencies in the frequency domain correspond to the average gross level of information in an image, whereas the higher frequencies correspond to edges, noise, and more detailed information.
Typically, images by nature are smooth and that is why most images can be represented using a handful of DFT coefficients and all of the remaining higher coefficients tend to be almost negligible/zeros.
This is is very useful in image compression, particularly for Fourier-sparse images, where only a handful of Fourier coefficients are required to reconstruct an image, hence only those frequencies can be stored and others can be discarded, leading to high compression (for example, in the JPEG image compression algorithm a similar transformation, Discrete Cosine Transform (DCT) is used. Also, as we shall see later in this chapter, filtering with the DFT in the frequency domain can be much faster than filtering in the spatial domain.