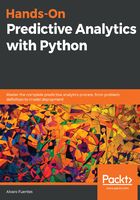
Communication and/or deployment
Goal: Use the predictive model and its results.
Finally, the model has been built, tested, and well evaluated: you did it! In the ideal situation, it solves the problem and its performance is great; now it is time to use it. How the model will be used depends on the project; sometimes the results and predictions will be the subject of a report and/or a presentation that will be delivered to key stakeholders, which is what we mean by communication—and, of course, good communication skills are very useful for this purpose.
Sometimes, the model will be incorporated as part of a software application: either web, desktop, mobile, or any other type of technology. In this case, you may need to interact closely with or even be part of the software development team that incorporates the model into the application. There is another possibility: the model itself may become a "data product". For example, a credit scoring application that uses customer data to calculate the chance of the customer defaulting on their credit card. We will produce one example of such data products in Chapter 9, Implementing a Model with Dash.
Although we have enumerated the stages in order, keep in mind that this is a highly iterative, non-linear process and you will be going back and forth between these stages; the frontiers between adjacent phases are blurry and there is always some overlap between them, so it is not important to place every task under some phase. For instance, when dealing with outliers, is it part of the Data collection and preparation phase or of the Dataset understanding phase? In practice, it doesn't matter, you can place it where you want; what matters is that it needs to be done!
Still, knowing the logical sequence of the stages is very useful when doing predictive analytics, as it helps with preparing and organizing the work, and it helps in setting the expectations for the duration of a project. The sequence of stages is logical in the sense that a previous stage is a prerequisite for the next: for example, you can't do model evaluation without having built a model, and after evaluation you may conclude that the model is not working properly so you go back to the Model building phase and come up with another one.